The rapid adoption of Generative AI (GenAI) tools, such as ChatGPT, has transformed various sectors, including marketing, legal, and software development. However, this rapid integration brings challenges, such as managing critical data access, mitigating costs, and ensuring compliance. To address these complexities, enterprises need to upgrade their data center management with an AI Data Fabric Copilot.
The Rise of AI Data Fabric
AI data fabric represents the entire path from application to data and back, integrating diverse components like storage devices, networking devices, data fabrics, CPUs & GPUs, Kubernetes & scheduler add-ons, databases, and cloud & datacenter environments. This comprehensive approach ensures seamless data flow and optimized performance across all AI infrastructure components.
What is a Data Fabric?
Gartner defines data fabric as an integrated layer of data and connecting processes1. It leverages continuous analytics over existing, discoverable, and inferenced metadata assets to support the design, deployment, and utilization of integrated and reusable data across all environments, including hybrid and multi-cloud platforms.
Data fabric is a comprehensive network architecture designed to facilitate high-speed, redundant, and resilient data transfer across distributed locations and systems. It goes beyond serving as the connective tissue between infrastructure components; it captures and orchestrates the seamless flow of data between each element. Akin to the internet’s multiple pathways that prevent single points of failure, a data fabric ensures reliable and uninterrupted data movement, providing organizations with a robust and flexible data integration backbone.
To ensure optimal performance, organizations must first leverage a robust data integration backbone that effectively manages metadata and maintains comprehensive knowledge of the infrastructure and data flow dynamics. This metadata, encompassing all aspects of data and pipelines, needs to be meticulously stored and maintained. Secondly, organizations must cultivate a deep understanding of their infrastructure and data flow patterns, enabling more efficient utilization and optimization.
Lastly, a resilient data integration backbone serves as the foundation upon which the data fabric operates, enabling the seamless integration and movement of data across the organization’s distributed landscape. The data fabric should support various data delivery styles, such as ETL, streaming, replication, messaging, and data virtualization. It should also cater to IT and business users, ensuring seamless data integration and accessibility.
The Need for Monitoring Your AI Data Fabric
Managing an AI Data Fabric involves understanding and controlling diverse infrastructure components, whether on-premises, at the edge, or in the cloud. Effective monitoring provides visibility into performance, end-to-end telemetry, proactive problem detection, TCO analysis, and capacity planning. However, many enterprises lack suitable IT controls to achieve this level of observability, leaving them vulnerable to costs, performance issues, and compliance risks.
- Costs, Efficiency, and Reliability: Model training often requires high-performance clusters available in the cloud, while resource usage for inference and model tuning is lower and more suited for on-premises deployment. AI on the edge presents additional challenges for inferencing and training non-LLM models. Enterprises need an integrated view of their hybrid environment to optimize cost, performance, and overall ROI.
- Data Access and Compliance: Ensuring appropriate access controls and correlating critical data access with end-user activity is essential. Enterprises must balance the inventory of new, costly chipsets and manage budgets effectively to align with business objectives and regulatory compliance.
The Four Primary Benefits of AI Data Fabric Monitoring
Given the challenges and complexities just described, the demand for a copilot for AI data fabric to enable intelligent monitoring is increasing rapidly. What IT leaders are looking for in an AI data fabric copilot includes:
- Unified Visibility: Real-time insights into data flow and performance across the entire AI infrastructure.
- Optimized Performance: Proactive identification and resolution of bottlenecks to ensure smooth data flow and maximize efficiency.
- Cost Control: Strategic resource allocation and TCO analysis to optimize financial resources.
- Enhanced Compliance: Monitoring data access and ensuring adherence to regulations, reducing the risk of breaches.
In summary, you can convert your data fabric into a powerful tool for managing and optimizing data flow within your organization. The result? The effective monitoring of your AI data fabric allows you to make informed decisions based on the data from your proprietary intellectual property.
Conclusion
The future of AI data management lies in the integration and optimization of AI Data Fabric. By adopting a hybrid AI strategy along with an AI Data Fabric management layer, enterprises can unlock the full potential of GenAI tools while mitigating risks and ensuring optimal performance. This approach empowers IT and business leaders to navigate the complexities of AI adoption and achieve their desired business outcomes.
Ready to learn more? Read the Virtana AI Data Center White Paper to explore how to address the complexities of the AI data center and discover how to leverage AI Data Fabric for your enterprise.
Reference
Gartner. (11 May, 2021). Contributor: Ashutosh Gupta. Data Fabric Architecture is Key to Modernizing Data Management and Integration. Retrieved from Gartner.
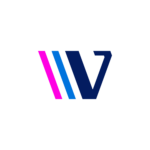
Shridhar Venkatraman
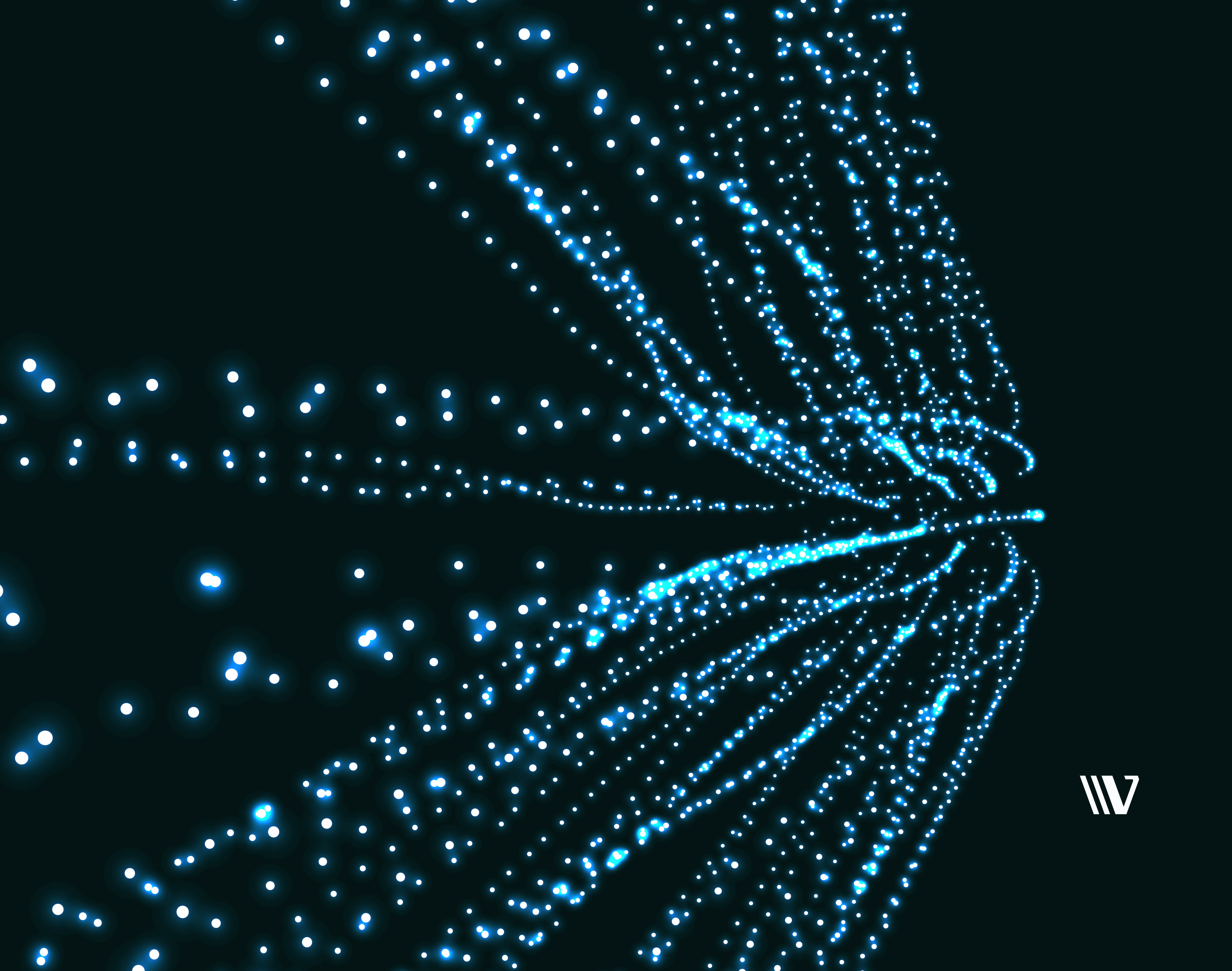