As organizations increasingly rely on complex and ephemeral infrastructure to drive business outcomes, the need for faster, more accurate, and automated IT operations has never been greater. Enter AIOps (Artificial Intelligence for IT Operations), a transformative approach that leverages AI and machine learning to automate and enhance IT operations management. These new learning systems can analyze massive amounts of network and machine data to find patterns not always identified by human operators. These patterns can both identify the cause of existing problems and predict future impacts. The ultimate goal of AIOps is to automate routine practices to increase the accuracy and speed of issue recognition, enabling IT staff to more effectively meet growing demands.
But how can you measure your AIOps capabilities and chart a course for continuous improvement? The answer lies in the AIOps maturity model.
What is the AIOps Maturity Model?
The AIOps maturity model is a framework designed to assess an organization’s current capabilities in AIOps and provide a roadmap for progressive enhancement. It outlines various stages of maturity, each representing a level of sophistication in applying AI and machine learning to IT operations.
The Stages of AIOps Maturity
There are five standard levels based on which maturity can be evaluated:
Level 1: Reactive
- Characteristics: At this stage, organizations typically have ad hoc processes heavily relying on manual interventions. Operations are primarily reactive, dealing with issues as they arise without a proactive approach.
- Capabilities: Basic monitoring tools are in place, but data analysis is manual and limited. Automation is minimal. They have siloed data and operations across various teams and tools.
- Challenges: High operational costs, frequent downtimes, and slow incident resolution are common issues.
Level 2: Managed
- Characteristics: Processes become more defined and structured. Though still largely reactive, there is a shift towards managing operations more systematically.
- Capabilities: Improved monitoring with centralized monitoring and better data integration. Some automated alerts and notifications are for predefined issues—initial use of root cause analysis capabilities for incident management.
- Challenges: Data integration is inconsistent, predictive capabilities are limited, and significant reliance on human intervention is still substantial.
Level 3: Strategic & Proactive
- Characteristics: Organizations standardize processes and start taking a proactive approach to problem management. Data integration improves significantly.
- Capabilities: Advanced monitoring with automated incident detection and initial deployment of machine learning models for anomaly detection. Predictive analytics begin to play a role. Also, improved collaboration across teams and automated incident response for common issues occur more regularly.
- Challenges: Ensuring data quality, integrating advanced analytics tools, and optimizing automation processes become key focus areas.
Level 4: Predictive
- Characteristics: IT operations become predictive, strongly emphasizing data-driven decision-making and significant automation.
- Capabilities: Organizations deploy advanced AI and machine learning models to predict incidents and performance issues. Automated root cause analysis and proactive problem resolution are key features. High level of automation across IT operations. Predictive maintenance and anomaly detection. Prescriptive recommendations for incident resolution.
- Challenges: Integrating disparate data sources, maintaining model accuracy, and continuously improving predictive capabilities are critical challenges.
Level 5: Prescriptive
- Characteristics: At the pinnacle of the maturity model, processes are fully optimized and automated with minimal human intervention. Continuous learning & improvement through machine learning feedback loops is the norm.
- Capabilities: Comprehensive use of AI for prescriptive analytics, real-time recommendations, and automated incident resolutions. Continuous integration and delivery (CI/CD) processes in place. Systems become self-healing.
- Challenges: Ensuring the robustness and reliability of automated systems, addressing ethical and governance issues related to AI, and adapting to constantly changing IT environments.
Let us fill out this table to evaluate the current AIOps maturity level in your organization:
If you score eight or below, you should develop more sophisticated alerting mechanisms, expand automation to more complex workflows & improve data integration, and explore predictive analytics.
If you score eight or below, you should develop more sophisticated alerting mechanisms, expand automation to more complex workflows & improve data integration, and explore predictive analytics.
If your score is between 8-16, then you should focus on improving the robustness of automated remediation processes, continue refining AI models for better root cause analysis, and expand context-aware automation across more areas of IT operations.
If you score over 16, you are already mature in AIOps practices and should continue to ensure continuous learning and improvement of AI models. Maintain alignment between IT operations and business goals & explore new opportunities for automation and AI application as technology evolves.
Give it a try yourself! Use the table below to score your organization’s current AIOps maturity level.
Business Benefits of Advancing AIOps Maturity:
- Improved Efficiency: Reduce manual workload and improve response times. AIOps enables real-time data analysis, which helps quickly identify and resolve issues before they escalate, reducing downtime and improving service availability.
- Enhanced Service Reliability: With AIOps, organizations can ensure higher service reliability and availability, leading to a better customer experience.
- Cost Savings: Lower operational costs through automation and predictive maintenance. By predicting and preventing outages, AIOps helps minimize downtime, which can be costly for businesses, particularly in critical systems.
- Better Decision Making: Use data-driven insights for strategic planning and operations. A proactive approach enables businesses to address problems before they impact operations.
Process for Implementing the AIOps Maturity Model
Assessment: Begin by evaluating your current processes, tools, and capabilities. Identify gaps and areas that require improvement.
Roadmap Development: Define clear goals for each maturity level and develop a step-by-step plan to achieve these goals.
Execution: Implement improvements(both tools & processes) in phases, regularly monitoring progress and adjusting the roadmap as needed.
Continuous Improvement: Regularly review and update processes and tools. Stay adaptable to new technologies and changing business needs.
Optimization: Aim for continuous improvement and optimization of IT operations.
Conclusion
Adopting and progressing through the AIOps maturity model enables organizations to systematically enhance their IT operations, reduce downtime, and improve overall efficiency and effectiveness. By leveraging AI and machine learning, organizations can transform their IT operations from reactive to proactive, predictive, and ultimately prescriptive, ensuring they are well-equipped to navigate the complexities of modern IT environments.
As you embark on your AIOps journey, remember that the goal is not just to reach the highest maturity level but to continuously adapt and improve, creating a resilient and responsive IT operation that can support your business’s evolving needs.
Are you ready to take your IT operations to the next level with AIOps? Share your experiences and insights in the comments below!
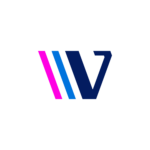
Meeta Lalwani
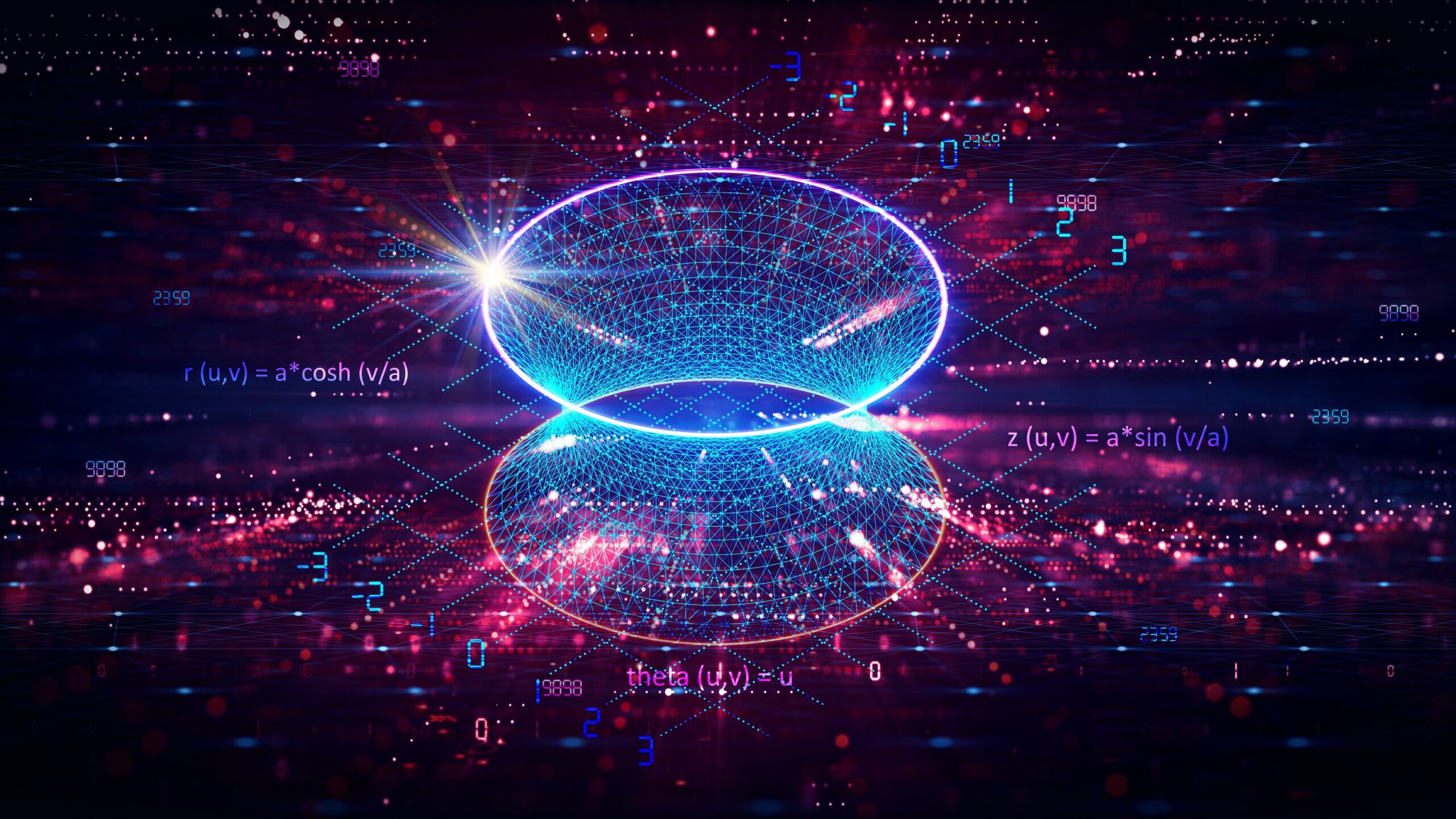