The rapid adoption of Generative AI (GenAI) tools like ChatGPT is transforming how businesses operate. These tools hold immense potential, impacting everything from marketing campaigns to legal research and software development. A recent study by PwC found that a staggering 54% of companies had integrated GenAI into their workflows as of November 2023 – a testament to the technology’s rapid adoption.
However, this rapid integration presents challenges for IT leaders. Managing these new AI services alongside existing IT infrastructure requires a strategic approach. This is where hybrid AI strategies come in, offering a win-win for both IT and business leaders.
Interested in learning more? Click here to get our whitepaper about how to address the complexities of the AI data center.
The Challenge: Managing Multiple Vendors, Multiple Sites, and Rising Costs in a Hybrid World
The hybrid approach to AI deployment recognizes the value of both on-premises and cloud environments.
- The cloud offers the scalability needed for intensive model training tasks.
- On-premises deployments provide greater control over data security, compliance, and resource management for inference and model tuning.
The availability of both cloud and on-premises environments gives IT and AIOps leaders unprecedented flexibility. For example, an organization can use Nvidia for their training systems and AMD for inferencing. That flexibility can often turn into a two-edged sword. Organizations using multiple clouds can choose the tools and applications best suited for their AI workloads. But that requires having the ability to support those clouds.
Managing this hybrid landscape requires careful consideration of several factors. The three most common hurdles include:
- Multivendor Management: How do you gain a unified view of your hybrid infrastructure to ensure seamless data flow and maximize performance?
- Multisite Visibility: How do you manage the data flow and ensure appropriate access controls across the hybrid environment?
- Cost Optimization: How do you allocate workloads between cloud and on-premises resources to optimize cost and performance?
The Hybrid AI Solution: The AI Data Fabric Copilot
The term “AI data fabric” is becoming commonplace amongst enterprises adopting hybrid AI strategies. The AI data fabric provides visibility across the entire pathway data takes, from the application to storage and back again. It encompasses various components:
- Applications managed by containers (Kubernetes) and GPU accelerators
- Data communication channels like PCI/NVMe and InfiniBand
- Storage systems like memory arrays, flash, and traditional HDD/SSD options
- Databases (both relational and NoSQL) that store and serve data
Managing this complex data fabric requires a comprehensive approach. This is where the AI Data Fabric Copilot comes in. It provides the tools and insights needed to:
- Gain Visibility: Understand how your AI Data Fabric is performing across all its components, both on-premises and in the cloud.
- Optimize Performance: Pinpoint bottlenecks and proactively address them to ensure smooth data flow and maximize performance.
- Control Costs: Analyze your total cost of ownership (TCO) and optimize resource allocation across the hybrid infrastructure.
- Ensure Compliance: Monitor data access across the entire fabric to ensure adherence to regulations.
The Benefits of a Hybrid AI Strategy for IT and Business Leaders
Embracing a hybrid AI strategy, supported by an AI Data Fabric Copilot, offers substantial benefits for both IT and business leaders. This approach provides a robust framework to manage the complexities of AI integration while optimizing performance, cost, and compliance.
For IT Leaders
Better Multivendor Management: The AI Data Fabric Copilot provides a holistic view of the entire AI infrastructure, integrating data from multiple vendors into a single dashboard. This unified perspective simplifies management, ensures seamless data flow across platforms, and maximizes performance while reducing integration complexities and downtime.
Enhanced Multisite Management: Monitoring data access ensures appropriate controls, maintaining data integrity and compliance while reducing the risk of unauthorized access and breaches. The AI Data Fabric Copilot provides real-time visibility into data flow and performance across multiple sites, enabling efficient management and coordination of operations.
Cost Optimization: By analyzing workload requirements and resource availability, IT leaders can make informed decisions about utilizing cloud or on-premises resources, optimizing TCO, and reducing expenses. The AI Data Fabric Copilot further enhances this by pinpointing performance bottlenecks and suggesting cost-effective solutions, ensuring financial resources are invested where they have the most significant impact.
For Business Leaders
Faster Time-to-Value: Utilizing cloud resources for development and testing enables rapid iteration and innovation, allowing business leaders to quickly deploy new AI solutions and stay ahead of market trends. The hybrid approach also allows businesses to efficiently scale inference tasks to meet varying demands without over-committing resources.
Enhanced Compliance and Risk Management: The AI Data Fabric Copilot assists with regulatory compliance by monitoring data access and usage across the entire infrastructure, reducing the risk of costly breaches. It also helps mitigate risks associated with data loss, security breaches, and operational disruptions by maintaining control over critical data and processes.
Optimized Business Performance: Comprehensive insights into AI infrastructure performance enable data-driven decisions that align with organizational goals. Streamlined operations and reduced data processing latency enhance workflow efficiency, improve customer experiences, and increase competitive advantage.
Conclusion
By implementing a hybrid AI strategy with an AI Data Fabric Copilot, enterprises can unlock the full potential of GenAI tools while mitigating risks and ensuring optimal performance. This approach empowers both IT and business leaders to navigate the complexities of AI adoption and achieve their desired business outcomes.
Ready to learn more? Follow this link to access our whitepaper, which further explores how to address the complexities of the AI data center.
Interested in optimizing your AI infrastructure? Contact us today for a personalized consultation and see how our solutions can transform your operations.
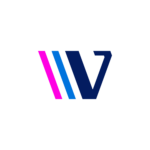
Shridhar Venkatraman
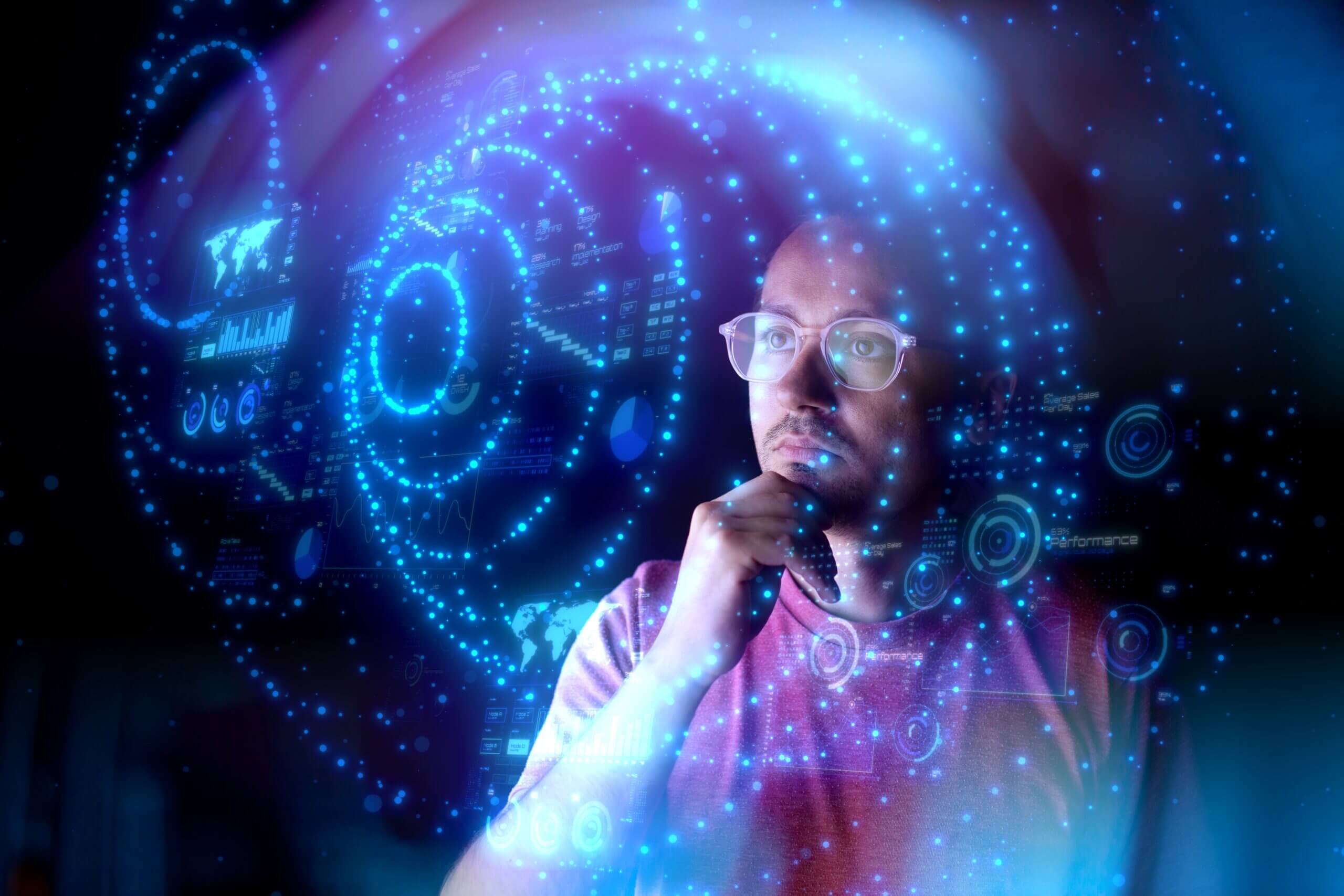