In the last few years, there has been a remarkable expansion in the benefits Artificial Intelligence (AI) offers. AI’s influence is pervasive everywhere, from voice-activated virtual assistants like Siri, Google Assistant, and Alexa to recommendation systems such as those employed by Netflix, Amazon, and Instagram and phone cameras that can provide real-time translation of text, signs, and menus. Nearly 77 percent of devices today use AI technology in one form or another.

Decoding AI Systems:
If we decode the world of AI, these systems are nothing, but potent computer setups trained on vast datasets, computing billions of attributes and delivering results in milliseconds. These robust machines are voracious consumers of electrical energy, akin to monstrous devourers. Moreover, they require copious amounts of water to maintain their cool. Studies predict that by 2026, data centers will guzzle an astounding 1,000 terawatts of electricity annually, nearly matching Japan’s total consumption. Trends show alarming spikes in water usage, with Google’s centers increasing by 20% from 2021 to 2022 and Microsoft’s by 34%. It is common knowledge that these resources are finite, and if data centers continue their extravagant consumption, scarcity looms on the horizon far sooner than anticipated. The MIT Technology Review reported that training just one AI model can emit more than 626,00 pounds (about 28,395 kg) of carbon dioxide equivalent – which is nearly five times the lifetime emissions of an average American car.
Despite these challenges, halting AI development is not a viable option. If businesses fail to leverage AI to deliver value, their competitors will step in and seize market share with better customer insights and more efficient, personalized products. However, sustainability concerns cannot be ignored. Many sustainability regulations have already been implemented in the European Union (SFDR), the United States, China, and many parts of the world, which will make it mandatory to commit to sustainable development.
Thankfully, there is already a concurrent initiative focused on reducing carbon footprints. CEOs ranked sustainability as the top challenge—ahead of regulations, cyber risks, and technology infrastructure. While this seems to conflict with the energy-intensive nature of AI, finding a middle ground is imperative. It is crucial to prioritize sustainability without sacrificing AI-driven growth. Many companies like Microsoft, Google, Apple, and Ford are already committed to being carbon negative.
Solutions to drive sustainable AI developments:
Developing AI models with a negative carbon footprint is undoubtedly challenging but not impossible. Here are some solutions that can be implemented easily to drive sustainable AI developments:
1. Measure & monitor current carbon footprint: The quote “You can’t manage what you don’t measure” was made famous by business management expert Peter Drucker. His point is that if you are not measuring and tracking your progress, what you are doing is not much better than guessing. Deploy an observability solution that can help you identify your current usage, CO2e emission, and provisioned servers and help optimize your resources. These tools can also help you gather actionable insights to optimize your carbon footprints.
2. Employ Narrow AI Engines: Train your model on smaller, less resource-intensive AI architectures that can deliver comparable effectiveness to larger ones. For instance, Microsoft recently introduced a language model trained on a curated library of textbooks, diverging from the typical vast corpus of internet text. Researchers claim this approach resulted in an AI proficient in specific domains exhibiting improved performance in certain tasks while demanding significantly lower power consumption. Practical examples of narrow AI engines are Chatbots, predictive analysis, image and speech recognition, and recommendation engines.
2. Utilize Efficient Computing Infrastructure: opt for energy-efficient hardware tailored specifically for AI workloads to markedly diminish the carbon footprint associated with both training and operating AI models. This encompasses utilizing low-power processors and maximizing server utilization. GPUs (graphic processing units) and TPUs (tensor processing units) are specifically designed to handle complex mathematical computations central to AI algorithms, offering significant computing speedups compared to traditional CPUs. Accelerated computing is energy-efficient computing.
3. Embrace Renewable Energy Sources for Powering AI Infrastructure: Offset carbon emissions linked to electricity consumption by harnessing renewable energy sources such as solar, wind, or hydroelectric power to sustain AI operations. Renewal energy sources are not only sustainable but are also approximately 60% cheaper compared to traditional energy sources. Amazon, Google, Meta, and Microsoft are some of the key global leaders committed to procuring renewable energy to power their facilities and thereby rein in their carbon emissions.
4. Enhance Data Efficiency: AI thrives on data, but we need to optimize the amount of data that needs to be stored to train AI models. Deduplication software, for example, can reduce the amount of data stored by more than 95%. Data compression is another technique. It reduces the number of bits needed to represent data. This helps in shrinking the data and lowers required storage units.
Streamline data storage and transmission processes to minimize energy usage, thereby playing a vital role in curbing the carbon footprint of AI models.
5. Implement Innovative Cooling Solutions: Traditional techniques for cooling IT (Information Technology) infrastructure, like fans and A/C, may not be enough to deal with the heat generated by today’s high-performance AI hardware. While not a water-based solution, liquid immersion cooling involves submerging servers or entire racks in a dielectric fluid or coolant. This method offers extremely efficient heat removal for energy-intensive AI workloads.
6. Use AI for Data Centers Optimization: AI can enhance infrastructure efficiency by providing immediate insights into energy and cooling use. A comprehensive review of industrial AI applications demonstrated that AI-supported HVAC (Heating, Ventilation, and Air Conditioning) systems can secure up to 25% in energy savings. By monitoring energy consumption and dynamically adjusting cooling and other parameters, AI can significantly reduce energy use in data centers and improve power usage effectiveness (PUE) metrics. For instance, Google saw a reduction of up to 40% in cooling energy consumption when they first implemented DeepMind’s machine learning algorithms in their data centers.
Conclusion:
AI will inevitably impact every human and enterprise on the planet. AI technology will grow, and so would be mandates around sustainability. While achieving a completely negative carbon footprint may be ambitious, implementing these strategies can significantly minimize the environmental impact of AI models. These strategies will help you to stay ahead of the competition without compromising faster AI adoption.
Author Bio:
Meeta Lalwani is a product management professional leading the AIOps (Artificial Intelligence for IT Operations) and AI-based Copilot Portfolio for the SaaS (Software as a Service) platform at Virtana. She is passionate about modern technologies and their potential to positively impact human growth.
References:
Hao, Karen: Training a single AI model can emit as much carbon as five cars in their lifetimes, June 6, 2019.
International Energy Agency (IEA): Electricity 2024, May 2024.
Matt O’Brien, Hannah Fingerhut, and the Associated Press, AI tools fueled a 34% spike in Microsoft’s water consumption, and one city with its data centers is concerned about the effect on residential supply, September 9, 2023.
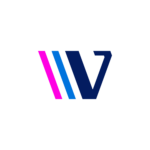
Meeta Lalwani
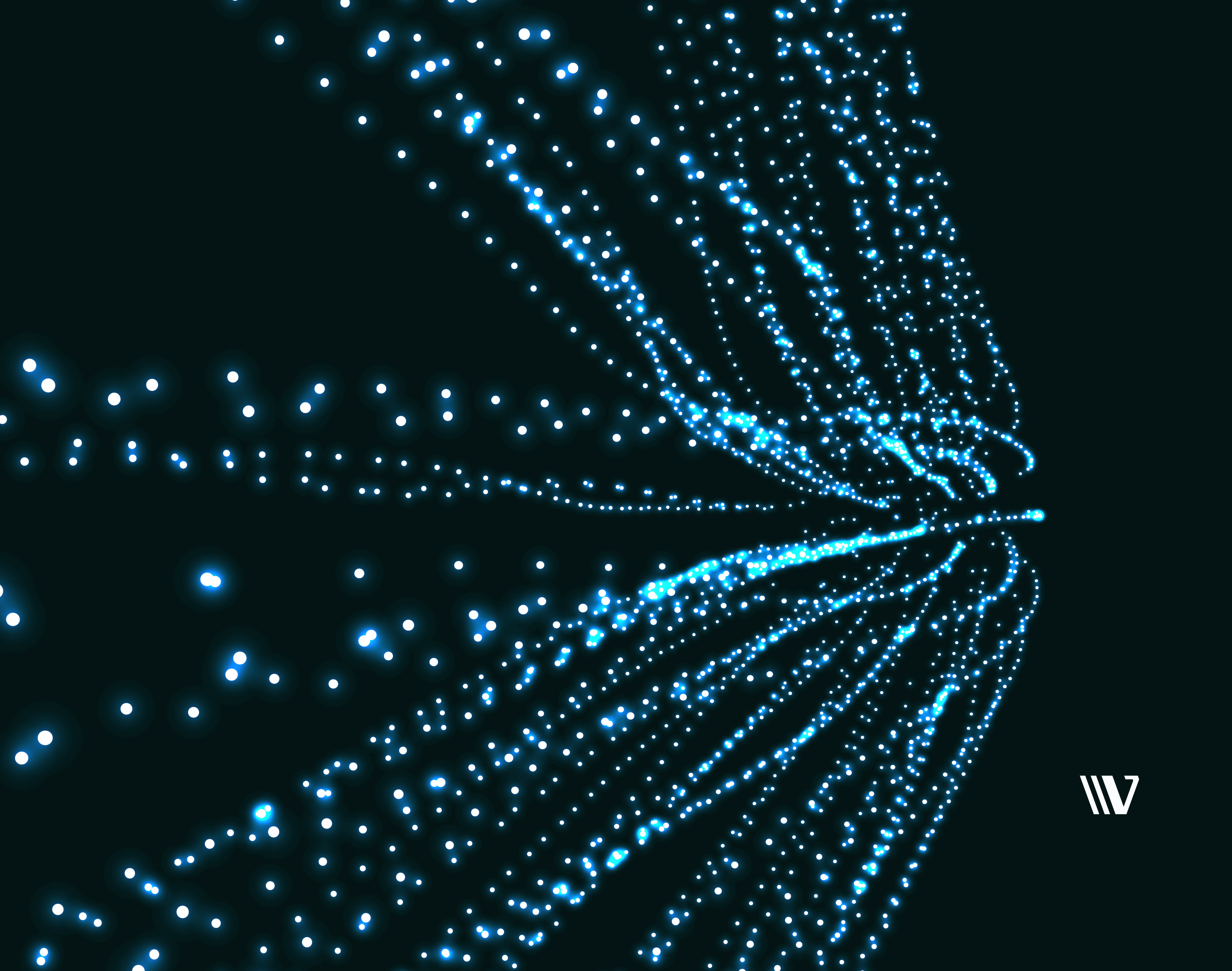